AI has been a game-changer in fraud detection within the financial sector. Traditional methods often relied on static rules and thresholds which were less adaptable to changing fraud patterns. AI, with its predictive modelling and anomaly detection capabilities, offers a more dynamic, efficient, and accurate solution. AI-based fraud detection systems can be used to detect a wide range of fraudulent activities, such as:
- Credit card fraud
- Identity theft
- Account takeover
- Money laundering
- Insurance fraud
By using AI to detect fraud, financial institutions can reduce losses, protect customers, and comply with regulatory requirements.
Effective fraud detection in the financial sector often involves a combination of the following AI techniques, which work together to provide comprehensive protection against a wide range of fraudulent activities. Incorporating AI into fraud detection systems offers financial institutions a robust and adaptable tool to guard against illicit activities. However, it’s essential to keep these systems updated, transparent, and overseen by human experts to ensure accuracy and trustworthiness.
Pattern Recognition: Supervised machine learning models can be trained on labelled datasets of known fraud cases and legitimate transactions. These models learn to recognize patterns and features associated with fraud, enabling them to classify new transactions as either fraudulent or legitimate. AI can recognize patterns in vast amounts of data. For example, if a particular pattern of transactions is indicative of fraud, the system can flag it for review.
Anomaly Detection: AI algorithms can analyze historical transaction data to establish a baseline of normal behaviour for each customer or account. Any deviation from this baseline is flagged as a potential anomaly. For instance, if an individual usually makes small transactions locally and suddenly makes large international transactions, the AI system can flag this as suspicious. Anomalies could indicate fraudulent activities such as unauthorized access, unusual transaction amounts, or atypical transaction locations.
Real-time Fraud Detection: Traditional systems often batch-process transactions, which means they might only check for fraud at specific intervals. AI can evaluate transactions in real-time, reducing the window in which fraud can occur. AI systems can also continuously analyze user behaviour and transaction patterns to create a dynamic profile of each account holder. Deviations from established behaviour profiles, like sudden large transactions or frequent changes in spending patterns, can trigger fraud alerts.
Predictive Analytics: AI-driven predictive analytics can forecast potential fraud trends based on historical data, allowing financial institutions to proactively adjust their fraud prevention strategies. By analyzing past fraud trends and combining various data points, AI models can predict future fraudulent activities.
Natural Language Processing (NLP): NLP techniques can be used to analyze text data, such as customer support chats or notes, to identify potential signs of fraud or fraudulent claims. AI can scan and analyze the text to detect deceit or attempts at evasion, especially in communication and customer service channels.
Geospatial Analysis: AI can check if the geographical location of a transaction aligns with the typical locations of a user. This can be particularly useful in detecting fraudulent activities stemming from unexpected regions or countries. As AI can analyze the geographic locations of transactions, it can compare them to the account holder’s typical locations to detect suspicious or unusual activity.
Network Analysis: AI can analyze network traffic and communication patterns to detect cyberattacks and unauthorized access to financial systems. By analyzing the relationships between different entities, be it individuals or institutions, AI can detect collusion or other coordinated fraudulent activities.
Biometric Verification: AI-based biometric authentication methods, such as facial recognition and fingerprint scanning, enhance security by ensuring that only authorized individuals can access accounts and perform transactions. AI can process and verify biometrics like voice, fingerprint, and facial recognition, providing an additional layer of security.
Graph Analysis: Analyzing the relationships between entities (e.g., customers, accounts, vendors) using graph analytics can uncover complex fraud networks and collusion. By understanding relationships between various accounts, AI can identify complex fraud schemes involving multiple entities and accounts.
Deep Learning: Neural networks, especially deep learning models, can recognize intricate patterns across vast datasets, which can sometimes elude simpler algorithms.
Self-learning Capabilities: AI models can continuously learn from new data. If a fraudulent pattern evolves or a new one emerges, the system can adapt without waiting for manual rule updates. AI systems can adapt and improve over time by continuously learning from new data and adjusting their fraud detection models accordingly.
Integrating Multiple Data Sources: AI can monitor transactions and interactions across multiple channels, including online banking, mobile apps, ATMs, and point-of-sale terminals, to identify cross-channel fraud schemes. AI can also incorporate external data sources, such as public records and blacklists, to enhance fraud detection capabilities.
Data Visualization: AI can assist in visualizing complex data patterns, making it easier for human investigators to understand and act upon potential fraud threats
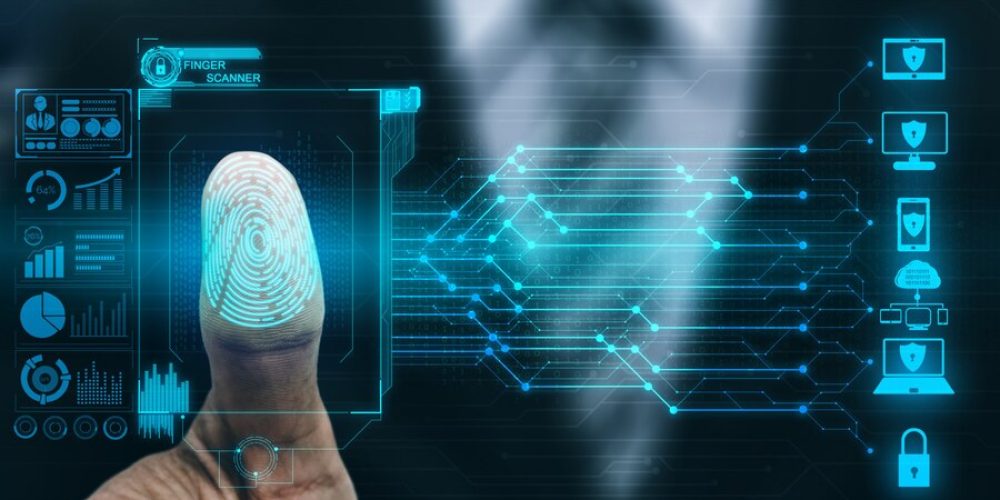
Firms need to be aware of the following challenges:
Data privacy: Handling customer data with care is paramount, and all AI systems must adhere to data protection regulations.
False positives: Overzealous systems might flag legitimate transactions as fraudulent, leading to customer dissatisfaction.
Complexity: Deep learning models, while powerful, are often termed “black boxes” due to their opacity, which might pose challenges in explaining decisions, especially in regulatory contexts.
In addition to detecting fraud, AI can also be used to prevent fraud. For example, AI can be used to:
- Personalize risk assessments for individual customers
- Develop adaptive authentication methods
- Educate customers about fraud risks
By using AI to prevent fraud, financial institutions can make it more difficult for fraudsters to succeed. Overall, AI is a powerful tool that can be used to detect and prevent fraud in the financial sector. By using AI, financial institutions can reduce losses, protect customers, and comply with regulatory requirements.